Data Annotation Reviews: Everything You Need to Know
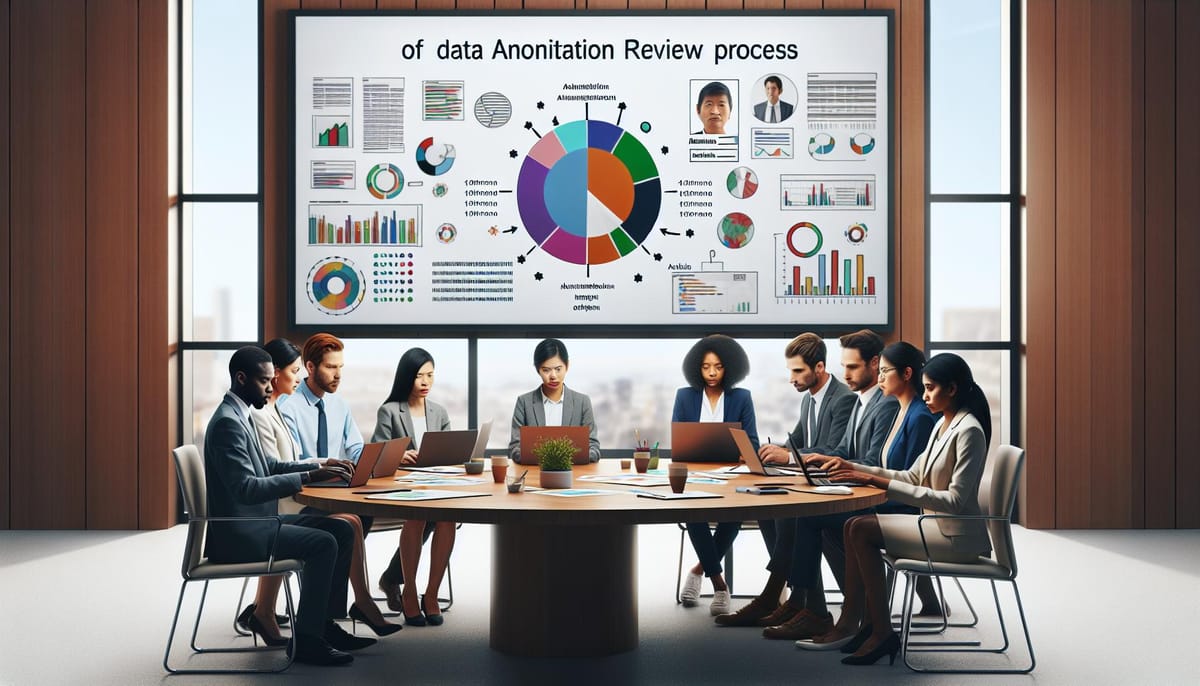
The foundation of machine learning and artificial intelligence is data annotation, which guarantees models are trained using relevant and accurate information. To keep quality and uniformity, nevertheless, the process calls for a careful inspection rather than finishing with labeling. Being very involved, I understand how important this is in perfecting data for best performance.
Examining annotated data guarantees that every classification and label follows the policies, not only helps to find mistakes. Reviewers are essential in bridging the gap between raw annotations and usable data since tools and procedures meant for accuracy help to close this difference. Though it's a rigorous process, it's what ensures consistent artificial intelligence results.
One cannot emphasize the need of quality control in data annotation. The evaluation process guarantees the data satisfies the highest criteria whether it comes to changing annotations, rejecting discrepancies, or including perceptive comments. This interesting yet demanding duty will help to define the direction of artificial intelligence evolution.
Understanding Data Annotation Tech
The foundation of artificial intelligence is data annotation technology, which provides the labeled datasets needed for training methods. Among the several chores involved in this process are image annotations, text tagging, audio transcription, and video labeling. Annotation of photographs, for example, requires the identification of objects or face traits; text annotation might mark entities or examine attitudes. These jobs guarantee that artificial intelligence systems generate correct predictions and identify trends.
Both manual and semi-automated approaches are used; annotators use specific tools for effectiveness. Platforms providing these features usually include tools customized to particular data kinds, therefore guaranteeing accuracy. Accurate annotations are essential since improperly labeled data impairs artificial intelligence's capacity for operation. Integration of advanced quality control techniques including reviews and corrections helps to keep annotations reliable. Data annotation is still essential for enhancing machine learning performance and application efficacy even if artificial intelligence technologies are becoming more and more important in many different sectors.
What is Data Annotation Tech?
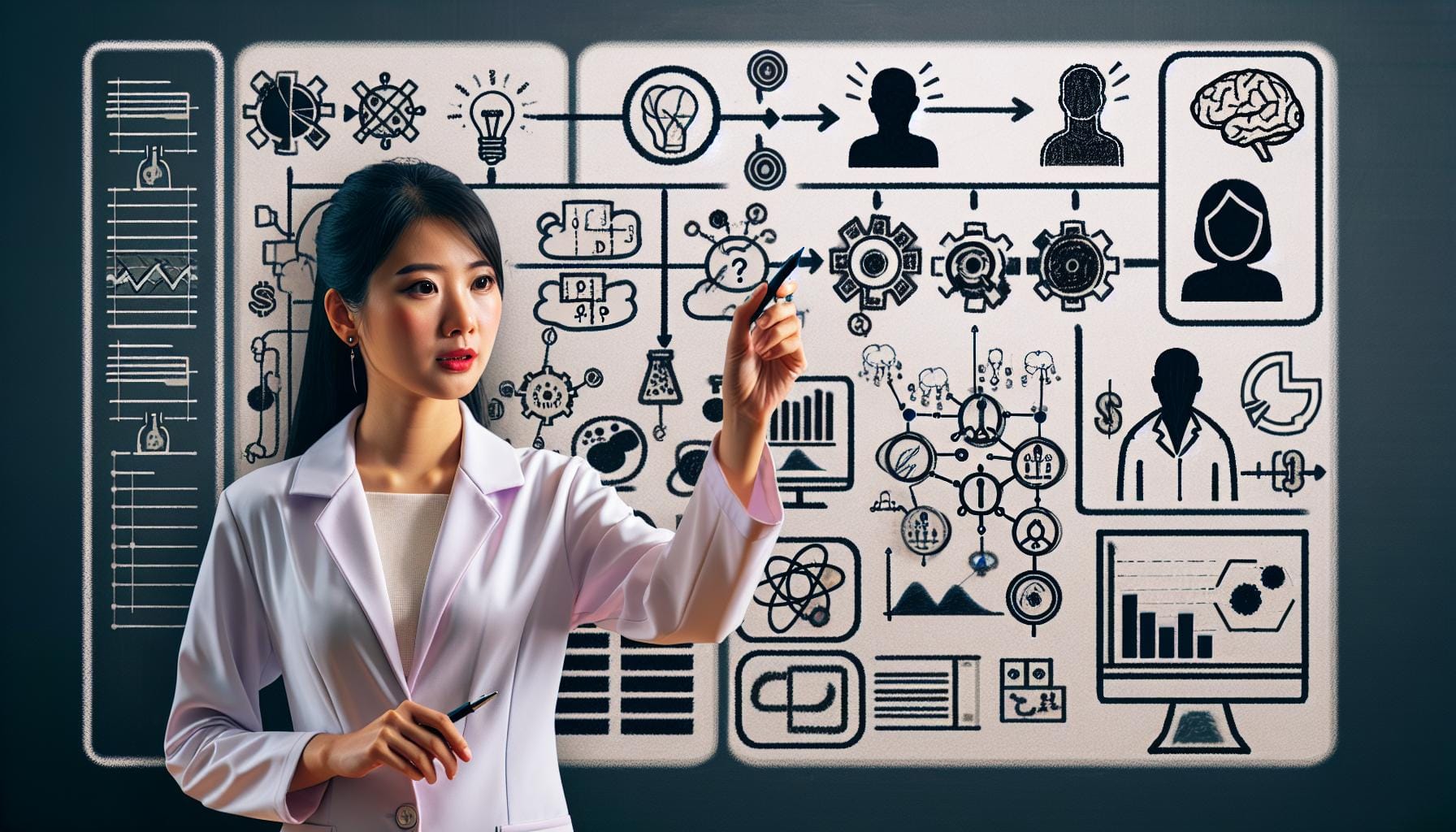
Data annotation technologies are the specific method of categorizing data to train artificial intelligence and machine learning algorithms. It entails adding relevant data to unprocessed datasets so that artificial intelligence systems may properly understand and act on input. AI models cannot detect patterns, make judgments, or produce correct results without this fundamental step.
The technique covers image annotations—where objects or faces are found using bounding boxes—text annotations involving labeling items or feelings, and audio annotations for transcribing speech or marking particular sounds. By naming events or scenes frame by frame, video annotation adds still another level. These jobs call for strong tools and different approaches to fit particular data kinds.
Commonly used manual or semi-automated annotation techniques are usually backed by systems including built-in quality control mechanisms. Not only improves AI performance but also affects sectors such virtual assistants, driverless cars, and predictive analytics by accurate data annotations.
The Role of Data Annotation Tech in AI Development
By offering annotated datasets needed for model training, data annotation technologies helps artificial intelligence. It helps algorithms to recognize objects in photos, interpret speech in audio, and sentimentally or contextally assess text. Driving systems like autonomous cars, language translating, and predictive analytics, this process supports developments in artificial intelligence.
Good annotations guarantees that artificial intelligence can learn precisely. Jobs such categorizing video frames, transcribing voice, and tagging items in text need for accuracy and domain-specific knowledge. We combine human intuition with machine efficiency using both automated tools and hand techniques.
Directly affecting AI performance is quality annotation. Unlabeled data runs the danger of misinterpretation, therefore compromising system integrity. Annotation reviews improve dataset quality, correct tags, and point up discrepancies.
Across sectors, trusted systems and annotators guarantee consistent, accurate datasets. This basis lets artificial intelligence grow and supports uses like financial forecasts, voice assistants, and healthcare diagnostics to operate as they should.
Legitimacy of Data Annotation Tech: Myths vs. Reality
Training AI and ML models depends much on data annotation technology, however doubts about its validity continue. Mostly this results from false information and the industry's lack of openness. Legitimate platforms guarantee credibility by following exact payment procedures, thorough policies, and easily available support systems. Verifying a company's validity depends much on reviews on sites like Trustpilot.
Driven by applications ranging from self-driving cars to voice assistants to predictive analytics, the sector is vital for fostering artificial intelligence creativity. It generates employment worldwide and provides a stable revenue stream together with flexible working schedules. Together, automated tools and human reviewers provide accurate, context-rich annotations.
Inconsistent rules and untrustworthy platforms claiming large earnings cause problems. Although reports of making $20 per hour abound, they do not reflect all possible employment. Researching platforms and assessing comments guarantees users can interact with trustworthy, legal services supporting artificial intelligence developments.
Is Data Annotation Tech Legit?
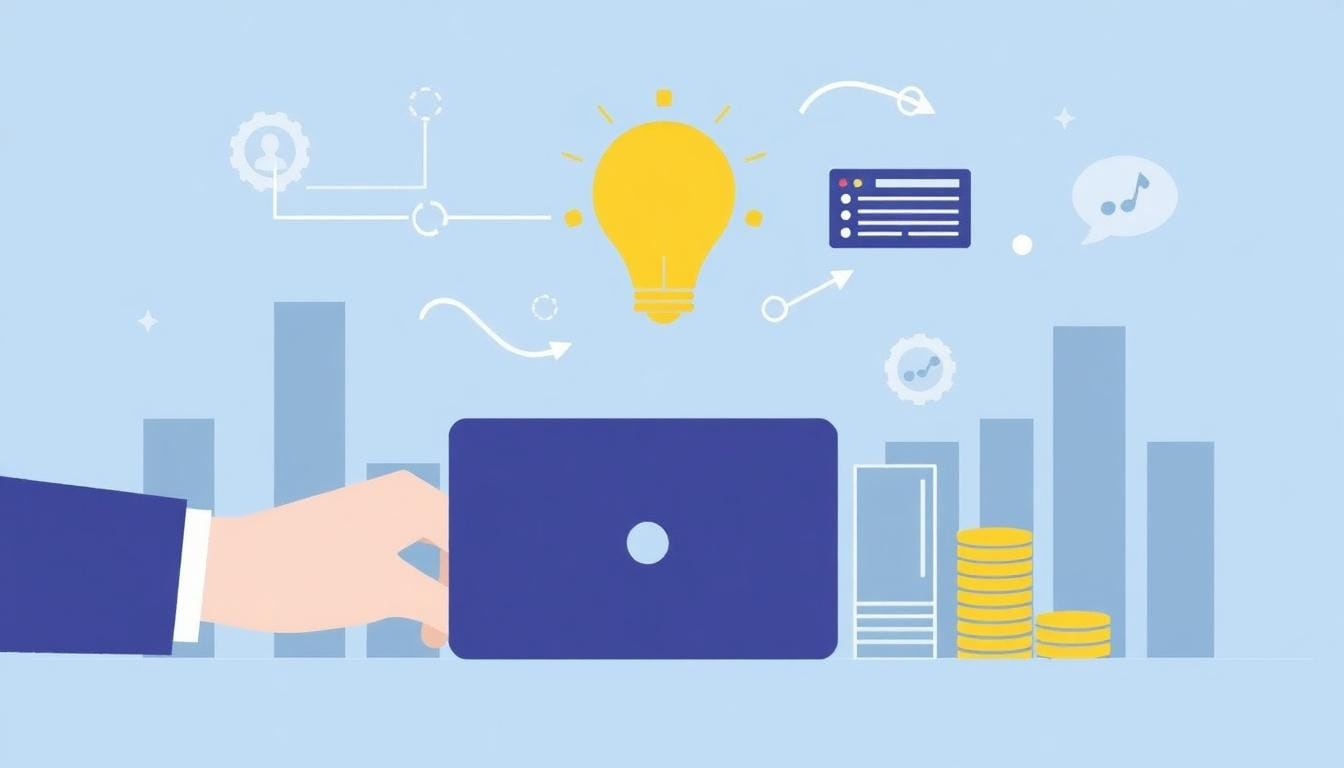
Advancement of artificial intelligence and machine learning depends on valid and important data annotation technologies. It guarantees the building of labeled datasets enabling algorithms to learn, adapt, and execute tasks including image recognition, speech processing, and decision-making. AI systems could not operate as they should without correct annotations.
Reputable sites providing data annotation tools keep openness by means of transparent pricing terms, easily available assistance, and thorough task instructions. Reputable platforms offering steady work possibilities include established organizations like Lionbridge and Amazon Mechanical Turk. Some less reliable platforms, especially in the freelance economy where openness can fluctuate, could also exist, though.
Researching company reviews on reliable websites like Glassdoor and analyzing user comments helps one to verify validity. Credibility is established in part by assessing their payment delivery record and upholding of fair policies. Safe practices help to lower possible dangers by means of safe routes of communication and documentation of finished work, thereby guaranteeing the validity of data annotation services.
How Does Data Annotation Tech Operate?
Raw data is ready for successful training of artificial intelligence systems by data annotation technologies. Data preparation and cleaning—where irrelevant, duplicate, or missing information is addressed to ensure consistency and quality—begin the process. There is standardizing of forms and filling in or elimination of missing data.
Data labeling and annotation occurs once ready to provide relevant tags or labels to datasets. Manual annotation—where human annotators label items, attitudes, or patterns following certain guidelines—is part of this process. Though quite accurate, hand annotations are labor-intensive. Automation can augment this approach by employing tools to speed labeling while lowering repetitive work.
The data type determines the several annotations techniques used. Bounding boxes for image annotations, text annotation tagging phrases, audio annotation transcribing of speech, and object tracking video frame labeling are among the examples. By means of reviews and updates, advanced quality control systems guarantee that annotations satisfy high criteria, thereby directly improving the performance of AI models.
Company Insights: Unpacking DataAnnotation Tech
DataAnnotation Tech is unique in that it provides remote possibilities where employees create their own schedules using a flexible work paradigm. Simplifying project submissions, tasks are available via a clean web interface. Designed largely to support machine learning and artificial intelligence models, projects include data labeling, chatbot interactions, and survey-based tasks.
Task complexity determines earning variation. Usually earning $20 or more hourly, annotators may find more pay incentives from specialized work. For regular donations, reported incomes run up to $14,000 annually. Some monthly income, like $3,200, show the platform's potential as a major extra source of income.
Still major issues are trust and validity. Mixed user comments highlight its need of clear payment systems and direction. While some employees mention task clarity problems, others report seamless payments. Investigating comments on websites such as Trustpilot guarantees informed interaction. The platform's wider industry influence is shown by its part in artificial intelligence training.
Who Owns DataAnnotation Tech?
DataAnnotation Tech's ownership is still unknown since no official declaration or records validate it. Some users hypothesize that may be a Surge AI subsidiary because of feature and platform structure similarities, although this link is unverified. Following a strategy shared by smaller or privately held digital firms, the company has not made public disclosure of its parent company or ownership.
Though the confusion, comments point to validity. Many times demonstrating operational dependability, users report continuous payments and task availability. Mixed reviews point to variation in user experience by highlighting problems such payment delays or unclear task directions.
Investigating the ownership of the platform is difficult without open communications. Reviewing Trustpilot or Glassdoor helps one confirm its credibility and help negotiate its reputation. Although ownership information can still be elusive, users should give top priority to evaluating the general dependability and validity of the platform prior to interaction.
Is DataAnnotation Tech a Good Company?
Though its ownership is yet unknown, DataAnnotation Tech is usually seen as a suitable platform for data annotation activities. Consistent payments and timely work availability reported by many users point to operational dependability. The technology is versatile in scope since it supports several annotation activities including legal data analysis, image categorization, and language model training.
Given workers pay between $5 and $15 per hour depending on task complexity, payment fairness is typically underlined. Reviewing Glassdoor and Trustpilot reveals good experiences include working from home and schedule flexibility. A few consumers do, however, bring up issues such delayed payments or unclear task instructions.
Reliability of the company depends on verification by several reviews and reliable sources. Although DataAnnotation Tech provides flexible work schedules and reasonable pay, occasionally problems with assignment submission and management communication surface. Reviewing material guarantees informed participation.
Earning Potential: How Much Can You Make with Data Annotation Tech?
Usually ranging from $10 to $20, data annotation roles provide flexible earning opportunities. Task complexity and platform will determine whether experienced annotators can surpass $20 per hour. For example, working four hours daily at $20 per hour comes out to be $80; eight hours comes out to be $160 in one day.
One can find really notable weekly earnings. One person said they made $852.58 working on a respectable platform after a week of intensive effort. Platform rates and annotator productivity impact monthly incomes ranging from $500 to $2,000.
Earning also influences task specialization. Often times, text tagging or sentiment analysis pays less than video annotations needing more accuracy. Top-notch systems draw experts with their continuous reimbursements, variety of duties, and help of qualified annotators. These earning trends show the possibility of data annotations as a rich distant income source for artificial intelligence research.
How Much Money Can You Earn from Data Annotation Tech?
Platform, task difficulty, and effort all affect the earnings in data annotations. Hour fees between $20 and $31 are available on sites like DataAnnotation.tech. Potential income at 464 part-time hours yearly at an average rate of $23 per hour comes to $1,938. Working two hours every day at $20 per hour consistently yields $14,600 yearly.
Task and platform determine monthly compensation ranges ranging from $500 to $2,000. Payment options generally include PayPal, and on certain systems withdrawals happen every three days. Higher rates can come from specialized annotations like video labeling.
Scheduling with flexibility helps employees to fit this employment to their daily habits. Task availability can vary, though, which would result in different pay. Selecting reliable platforms and keeping high-quality output guarantees stability in income and productivity.
Does Data Annotation Tech Really Pay?
Indeed, respectable data annotation systems pay, usually by direct transfer or PayPal using dependable techniques. For entry-level work, hourly pay for U.S. annotators typically vary from $10 to $20; depending on task complexity, expert specialists can make up to $70 per hour. Average yearly pay range from $38,353 to $48,241; hourly pay vary from $21. Based on the platform, payment schedules vary; weekly, bimonthly, or monthly possibilities are available.
Administrative procedures cause sometimes payment delays on platforms. Most problems are resolved by quickly getting customer support. Reviews from reliable websites such as Trustpilot and Glassdoor point to conflicting experiences; some customers cite on-time payments and work clarity, while others note delays or confusing directions. Researching platforms and knowing upfront payment terms can help to guarantee a flawless encounter. For both fresh and seasoned annotators, data annotation provides flexible income sources.
How Long Does Data Annotation Tech Take to Pay?
Payment available from DataAnnotation Tech is hourly or per assignment. Earnings for hourly projects become withdrawable seven days following logged time worked. Funds for per-task projects become available for withdrawal three days later. Once the dashboard's "Transfer Funds" option is clicked, PayPal payments are instantaneous.
While frequency of withdrawal is not limited, many contributors would rather have weekly withdrawals for simpler financial tracking. On some systems, administrative delays cause inconsistent payment times. Task difficulty and platform dependability affect earnings usually ranging from $5 to $15 per hour. Knowing certain payment schedules guarantees proper financial control.
Job Viability: Is Data Annotation a Good Career?
Particularly in fields depending on artificial intelligence and machine learning, data annotation shows great employment possibilities. Growing artificial intelligence use is expected to fuel the $3.5 billion AI and ML data labeling industry by 2024. For specialists in disciplines such government programs, medicine, and finance, this need generates consistent opportunities.
To support their artificial intelligence projects, several businesses designate data annotators. While specialist tasks like annotating medical data or sentiment analysis have more earning possibilities, entry-level roles offer an easily available beginning point. Depending on task complexity and platform, average monthly earnings fall between $500 and $2,000.
Many times, data annotation is seen as a necessary step toward artificial intelligence. It provides useful knowledge for moving into advanced positions like data science or artificial intelligence development. Human supervision is still absolutely vital for advanced annotations, which guarantees long-term relevance in the sector even if the labor can be contractual and repetitious.
Is Data Annotation a Good Career?
Particularly in artificial intelligence and machine learning, data annotations provide a bright future job path. By generating correct, labeled datasets, experts in this domain significantly help to train AI models. Projected to be $8.22 billion by 2028, the worldwide data annotation market shows the strong demand for this knowledge.
Jobs in this field range from healthcare to banking, driverless cars to natural language processing. While specialist tasks like annotating medical or video data pay more, entry-level jobs offer easily available chances for remote employment. For most positions, monthly income falls between $500 and $2,000; for more difficult tasks, experienced annotators earn even more.
Though it can seem monotonous, labor gives people basic information that will help them move into sophisticated artificial intelligence positions. For artificial intelligence systems, data annotation is still absolutely vital since it generates employment constantly and provides a major starting point for jobs in the technological industries.
Is Working for Data Annotation Tech Worth It?
Working for DataAnnotation Tech pays reasonable rates, provides flexibility, and paid training. Many reviewers value the flexibility to work remotely and chose projects related to their hobbies. Usually ranging from $5 to $15, hourly rates vary depending on skill and complexity of work like sentiment analysis or video annotations. Some users say they make up to twenty or more dollars an hour.
Projects involving picture labeling, transcription, and text tagging cover a variety of disciplines, hence they are usually interesting. One drawback of the boredom of repeated chores, though, can be Common concerns are payment delays and unclear job standards; nonetheless, many employees claim prompt pay and consistent support. Generally speaking, it's a decent choice for part-time or extra income—especially for individuals hoping to get expertise in artificial intelligence. Careful investigation of platform validity guarantees a better experience, thereby preventing possible problems with income or task clarity.
Ease of Work: What is Data Annotation Tech Really Like?
Flexibility specifies data annotation tech jobs and lets remote work and customized times possible. Often choosing activities related with their experience, annotators can operate from any location with internet access.
Repetitiveness, though, defines numerous jobs include classifying photos, transcribing sounds, or tagging text data. Particularly for easier tasks like bounding box annotations or rudimentary speech transcription, constant repetition might cause monotony.
Task availability differs depending on the platform; gaps create different possibilities. While some systems guarantee consistent processes, others suffer demand variations that affect income.
Earning potential follows task complexity and skill level. While specialist annotations like medical data or sentiment analysis can top $20 hourly, simple jobs might pay $10–$15 hourly.
Annotations call for tools and instruction. Modern systems offer comprehensive instructions, therefore reducing mistakes and raising accuracy. Crucially for satisfying client standards, feedback loops and quality control systems improve annotator performance.
Is Data Annotation Easy?
Data annotation is far from always simple since it entails difficult and time-consuming activities. Especially for complex projects, manually annotating vast datasets is labor-intensive and calls for annotators to thoroughly analyze and identify data. For object tracking or medical data labeling, for which considerable knowledge is needed, examples include video annotation.
Keeping consistency and quality poses still another difficulty. Errors or personal interpretations might compromise the dependability of annotations, therefore affecting the accuracy of artificial intelligence models. Review procedures exist to correct these disparities but increase the general burden.
Furthermore complicating the process are bias in annotations. Problems arise when datasets lack diversity or human annotators inadvertently bring personal prejudices. These issues compromise AI efficiency and fairness, therefore restricting performance in practical uses.
Task-specific variation in annotation difficulty For example, sentiment analysis in text calls for a sophisticated knowledge while simple object classification in photos differs greatly. Easy of completion can be influenced by the platform, tools, and rules of use used.
What Kind of Work is Data Annotation Tech?
Data annotation technologies are those meant to label different kinds of data for artificial intelligence and machine learning systems. Image annotations—that is, bounding box-based object or feature identification—allow one to find traffic signs in datasets of self-driving cars. While audio annotation focuses on transcribing speech or categorizing background sounds, text annotation tags entities, sections of speech, or feelings to help artificial intelligence models interpret language. Often for use in surveillance or action recognition, video annotations mark objects or actions frame by frame.
Every job calls for different equipment and knowledge. While complicated annotations, such medical data labeling, required more knowledge, simpler chores like tagging terms in text may require little training. Many times, platforms offer precise rules to guarantee uniformity. While semi-automated approaches assist efficiently manage big datasets, manual methods guarantee accuracy even if they are repetitious. Across sectors including healthcare, banking, and transportation, these diverse projects together drive artificial intelligence advancement.
Application Process: How to Get Started with Data Annotation Tech
Signing Up
I first open an account on the chosen data annotation tool. To finish the registration, this stage consists in providing name, email, and work experience both personally and professionally.
Qualifications and Training
Once registered, platforms may assign training assignments. Doing these helps me decide whether I would be appropriate for tasks like voice transcription or image tagging. Accuracy in these chores raises the possibility of getting projects.
Project Selection
Projects show on my dashboard once training is over. Tasks range from text annotations for sentiment analysis or video labeling for object tracking to let me choose those fit for my background and hobbies.
How to Pass DataAnnotation Tech Test?
Preparation
Knowing the test criteria increases accuracy. To be sure what to expect, I go over all policies and directions. Familiarizing myself with particular test-related annotation tools such as RectLabel or Labelbox is absolutely crucial. I break apart the sample data and examples to grasp the assignments. Finding the required deliverables and output style guarantees that I follow the criteria of the test.
Understanding Guidelines and Techniques
Following the rules determines consistency. I go over them carefully to make sure my work satisfies standards of excellence. Examining example annotated datasets—such as bounding box annotations or labels for categorization—helps me work on different annotation tasks. Investigating segmentation projects helps me to manage several needs. Using this information guarantees that my grasp of technical procedures complements the objectives of the test.
Does Data Annotation Tech Have an Interview?
For most positions, Data Annotation Tech does not do conventional interviews. Rather, candidates finish qualifying exams meant to evaluate their competencies. Usually acting as the starting point, these exams are simple. Tasks could include, for instance, annotating text data according to particular rules or marking objects in photographs.
Specialized roles such as team leadership or quality control could incorporate extra tests or casual conversations to confirm knowledge in data annotation techniques. Entry-level positions concentrate on finishing examinations and paid qualifying phases, which assist to allocate projects appropriate for a candidate's qualifications.
Unlike usual hiring procedures, engagement with HR or management during first phases is rare. For remote workers particularly, this simplified approach guarantees effective and easily available onboarding. DataAnnotation Tech matches the choosing process with task-specific criteria by substituting skill-based assessments for interviews.
Availability and Accessibility: Where Can You Work for Data Annotation Tech?
Significant flexibility provided by data annotation tasks helps to fit remote and work-from- home settings. Locations are not limited since most jobs may be done anywhere with a consistent internet connection. Geographic diversity lets one engage from places like Columbus, Ohio, or Alpharetta, Georgia.
Remote roles rule the market. Most systems, like DataAnnotation Tech, offer completely remote possibilities so that employees may choose jobs best for their level of expertise. Many people, especially stay-at-home parents and students, gain from this flexibility.
In this field, part-time and freelance contracts are rather frequent. These choices help annotators create flexible timetables that follow personal obligations and pay for themselves. Task-based payments—which reward better-quality labor with bonuses—are common among companies.
Sites like Glassdoor compile user reviews to assess particular choices. Examining compliance, payment systems, and task clarity of a platform helps one determine its credibility and guarantees a safe and easily available annotation career.
What Countries is Data Annotation Tech Available In?
Globally operating and providing chances in many different areas, data annotation technology Leading the market is North America, where advanced technology adoption across sectors like healthcare, BFSI, and IT drives demand from the United States and Canada thanks to strong AI expenditures. Leading market players and large financing for artificial intelligence research help to explain this supremacy.
Data annotation services have quickest expansion in the Asia Pacific area. Thanks to sectors including e-commerce, automotive, and healthcare, nations including China, India, and Japan show great demand. Quick digital adoption and affordable labor help to hasten the growth of the area.
Many times catering globally, platforms offering annotation services have access dependent on data type, language, and regulatory restrictions. Many tools meant for remote work guarantee flexibility regardless of location. However, depending on operating areas or regulatory frameworks, limitations or particular criteria could apply; hence, the need of extensive platform research before involvement is underlined.
How Long Does It Take to Hear from Data Annotation Tech?
Hearing from Data Annotation Tech varies on the duration of their hiring procedures. Responses vary.
Career Growth: Is There a Future in Data Annotation?
Demand for artificial intelligence and machine learning will help data annotation to have great possibilities. By 2024 the market is expected to be $3.5 billion.
Salary Expectations: How Much Can You Earn in Data Annotation Tech?
Platform rates and task difficulty determine monthly profits between $500 and $2,000.
Work Environment: Pros and Cons of Working with Data Annotation Tech
While repetitious work and possible payment delays are drawbacks, the advantages include flexibility, remote alternatives, and good salary.
Legitimacy of Payment: Does Data Annotation Tech Actually Pay?
Indeed, Data Annotation Tech usually pays consumers; however, occasionally administrative problems cause delays.
Does Data Annotation Tech Really Pay?
Indeed, most users say they get their payments on schedule; although occasionally delays occur.
Is DataAnnotation Tech a Good Company?
Although for many people dependable, experiences differ based on payment and task clarity.
How to Pass DataAnnotation Tech Test?
Follow guidelines particular to your platform and carefully finish qualifying tests.
What Countries Is Data Annotation Tech Available In?
Though tasks are available wherever, platform and project needs determine availability.
How Long Does Data Annotation Take to Pay?
Usually paid weekly or monthly, platforms handle unexpected delays with help.
What is data annotation in AI and machine learning?
Data annotation is the act of identifying data—such as photos, text, audio, and videos—such that they could be used for training AI and machine learning models. It entails organizing and giving raw data relevant background so that artificial intelligence systems may identify trends and generate reliable forecasts.
Why is data annotation important in AI development?
Development of artificial intelligence depends on data annotation since it guarantees that machine learning models are trained on correct and high-quality datasets. This approach enhances AI's capacity to understand inputs, hence improving performance in jobs including sentiment analysis, image recognition, and speech processing.
How does quality control impact data annotation?
Quality control guarantees consistent, accurate, and project goal alignment annotations. Reviewing and fixing mistakes helps labeled datasets to become more reliable, therefore immediately improving the performance of AI models and avoiding problems brought on by inadequate annotations.
What tasks are involved in data annotation?
Image labeling (e.g., bounding boxes), text tagging (e.g., entities or attitudes), audio transcription, and video labeling (e.g., action tracking) are common chores in data annotations. Every choreis catered to the particular needs of the artificial intelligence project.
Can data annotation be automated?
Indeed, data annotation can speed up chores and cut repeating effort by using automated technologies. To guarantee accuracy, handle difficult annotations, and offer control to prevent dataset bias, human annotators are still nevertheless absolutely vital.
What skills are required for data annotation jobs?
Depending on the project, key talents for data annotation are attention to detail, expertise with data labeling technologies, a basic knowledge of artificial intelligence concepts, and occasionally proficiency in particular languages or subject area.
Is data annotation a legitimate industry?
Indeed, data annotation is a real and expanding sector that aids artificial intelligence developments in many different fields. Reliable chances abound on trusted sites like Lionbridge and Amazon Mechanical Turk; but, for authenticity, research platforms are crucial.
How much can you earn as a data annotator?
For entry-level work, data annotators usually make between $10 and $20 per hour; specialized professions pay up to $70 per hour. Task complexity and hours done determine monthly income; it ranges from $500 to $2,000.
Are data annotation jobs flexible?
Indeed, employment in data annotations are quite versatile. Mostly remote, they let people work from anywhere with an internet connection. Part-time and freelance jobs let employees choose their own calendars.
Is data annotation a good career path?
Particularly for those looking into the AI sector, data annotation offers a bright future job path. It provides basic knowledge for moving into sophisticated tech positions, and its increasing demand guarantees consistent employment prospects all around.